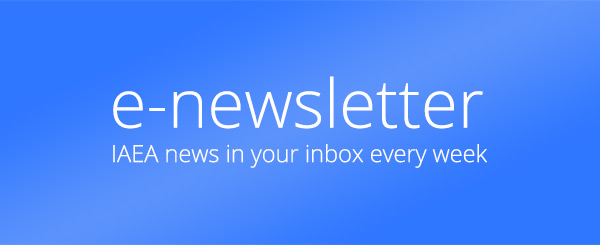
If you would like to learn more about the IAEA’s work, sign up for our weekly updates containing our most important news, multimedia and more.
The role of artificial intelligence and medical imaging in addressing zoonotic diseases
Georg Langs
Machine learning (ML), a growing part of artificial intelligence (AI), uses computers to solve complex tasks — not through programed solutions, but by creating models that can learn from examples. This approach has made great strides in recent years. ML models can now recognize people and objects in images; understand, translate and generate spoken language; and identify subtle relationships in biological data. As these models have grown more robust, they have become a part of our daily lives.
In health care, ML models play an increasingly important role in precision medicine, where they inform treatment decisions based on medical imaging data (e.g. computed tomography (CT), magnetic resonance imaging (MRI), positron emission tomography (PET) and X-rays), genomics and other clinical variables. By recognizing subtle patterns, disease progression signatures and treatment responses, the models can predict the risks and future course of a disease in individual patients. In medicine, ML models enable new measurements, quantitative observations and the discovery of novel knowledge about disease.
In lung diseases, ML models have identified new dynamic disease patterns linked to progression and response. With ML, subtle changes in the lung tissue and its imaging characteristics are measurable and turned into a new vocabulary of disease signatures. These signatures link a set of different observable image patterns reoccurring across the patient population. Their gradual change informs our understanding of the disease and allows us to predict individual risk with higher accuracy. Furthermore, ML integrates additional imaging information, capturing co-morbidities to improve prediction for individual patients.
ML is becoming a tool of research and discovery in medicine, as it makes distributed patterns tangible. Yet, while we understand prediction, we are still in the infancy of translating models into mechanistic representations of the biological processes underlying disease and treatment response. Here, intense progress can be expected in the coming years.
The role of medical imaging and machine learning
Medical imaging used together with ML offers a fine-grained view of the phenotypical variability of individual patients and their disease trajectory. It complements other observations, such as the characterization of viruses, genomic and epigenomic profiles, proteomics or laboratory findings, capturing systemic processes. Medical imaging data is acquired in tremendous quantities, as part of routine examinations. As our vocabulary for using this information grows, computational models afford us detailed insights into the variability of disease, new clinically relevant phenotypes and the relationship between individual patient, disease and treatment.
During the COVID-19 pandemic, only a few weeks after the causative pathogen was described, findings in chest radiographs and CT images were published and recognized as rather characteristic for COVID-19, in contrast to the relatively unspecific respiratory clinical symptoms. They carried unseen configurations, and, in the early phase of the pandemic, CT emerged as a diagnostic tool. As testing became available, its role changed from supporting diagnosis to informing treatment and management of individual patients.
This spells out the possible role of AI in the management of future zoonotic diseases transitioning into pandemics: the early detection of a novel disease phenotype in the clinical population and the early and effective guidance of patient treatment.
Potential and objectives of artificial intelligence: What if there are no examples?
The challenge of a zoonotic disease turning into a pandemic is that, on first sight, the paradigm of learning from examples seems to fall apart. We don’t have years of observations to teach a machine, and we don’t know what to look for when trying to detect a new disease or variant outbreak. This places the role of ML firmly in the area of discovery and identification of relationships observed in a heterogeneous patient population.
Firstly, the detection of anomalies — image patterns, their configurations or their co-occurrence with non-imaging findings — is picking up speed in the ML community. Methods such as generative adversarial networks can learn how imaging varies in a controlled population, so that the detection of novelty is becoming feasible, even if no labelled training data has been available. Currently, this is used to expand our vocabulary of markers, but it could also trigger the identification of novel emerging phenotypes.
Secondly, ML models could be an effective means to better understand the relationship between observed patient characteristics, disease course, and treatment needs and response based on early cases in the routine population before best practices are established. This could aid the identification of effective treatment and inform the creation of guidelines in the early phase of a pandemic.
Lessons learned: How to get there?
To enable the effective use of AI and imaging for future zoonotic diseases, we must overcome several obstacles. During the COVID-19 pandemic, barriers prohibiting the fast sharing of clinical (and imaging) data and its availability for the research community have had a crippling effect on the efficacy and robustness of the resulting ML models. We need to rapidly and transparently collect and curate such data globally to make it available to the research community. Shared data and benchmarks have a catalytic effect on the speed and quality of development.
On the methodological research side, we must advance our capability to deal with biases and confounders in data, stemming from the heterogeneity and diversity of the world. ML models don’t have to only repeat results but be fair models that separate biological relationships from sub-optimal and possibly biased treatment decisions. We must ensure that the training of models is based on inclusive data sets that benefit the vast population and does not exclude communities, regions or individual groups.
Finally, we must engage the ML community to ensure we support and inspire innovative minds around the world to tackle the challenges of turning observations into tools that help us to detect emerging zoonotic diseases earlier, as well as to better manage prevalent ones. The community can help find the best and most precise treatment schemes to serve individual patients and develop techniques to accelerate the development of novel and innovative treatments.
Georg Langs is the Head of the Computational Imaging Research Lab at the Department of Biomedical Imaging and Image-guided Therapy, Medical University of Vienna. A professor and researcher in artificial intelligence and its application in medicine, Langs is also affiliated with the Medical Vision Group at the Massachusetts Institute of Technology (MIT) Computer Science and Artificial Intelligence Laboratory.